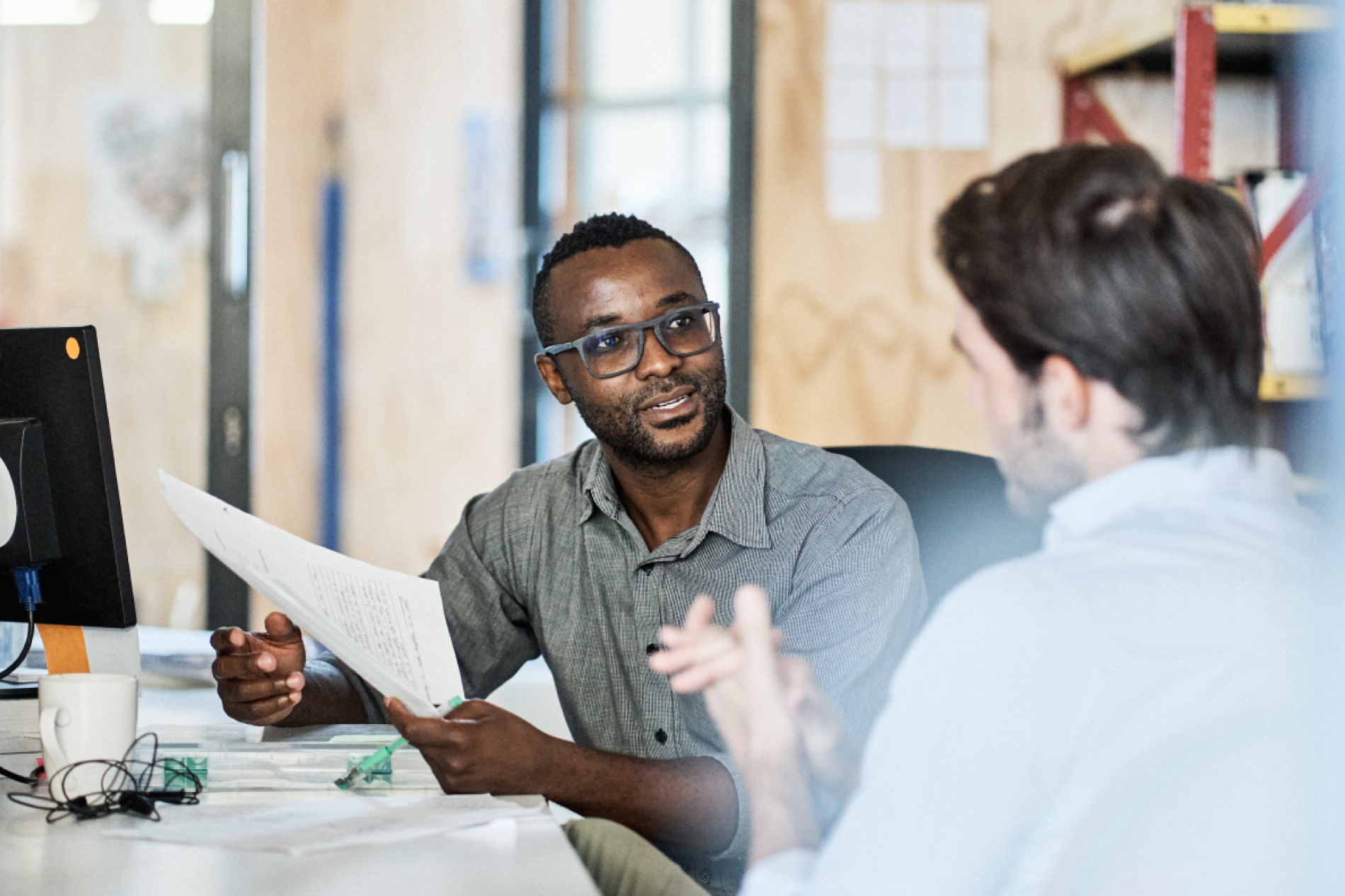
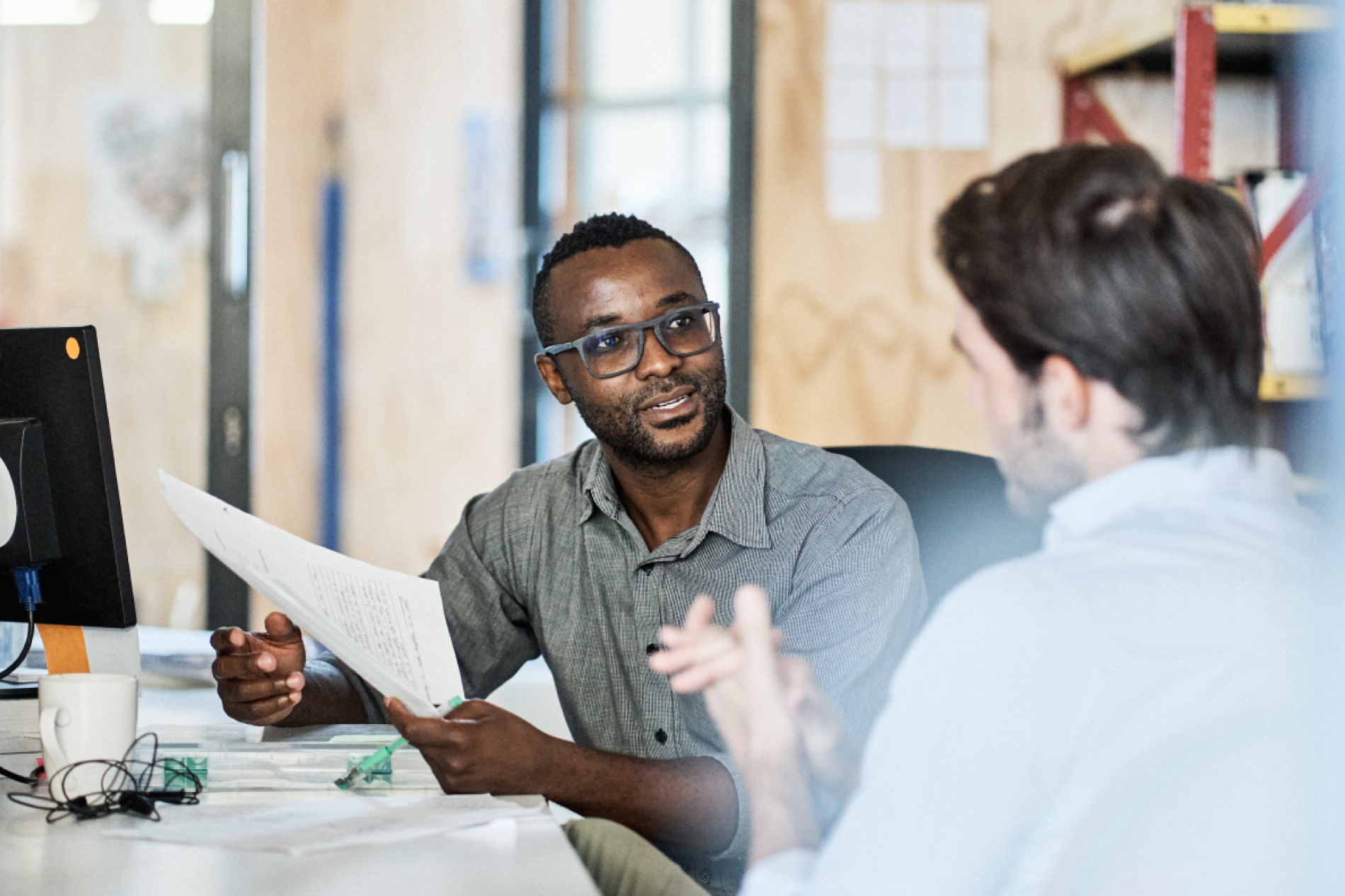
By Michael Kanellos, Head of Influencer Relations, Marvell
Bigger is better, right? Look at AI: the story swirls with superlatives.
ChatGPT landed one million users within five days,1 far surpassing the pace of any previous technology. The compute requirements of training notable AI models increases 4.5x per year while training data sets mushroom by 3x per year,2 etc.
Bigger, however, comes at a price. Data center power consumption threatens to nearly triple by 2028 primarily because of AI3. Water withdrawals, meanwhile, are escalating as well: by 2027, AI data centers could need up to 6.6 billion cubic meters, or about half of what the UK uses.4 The economic and environmental toll over the long run may not be sustainable.
Conceptually it is easier to understand how larger models translate into a "better and more capable" model. The more layers or parameters the models have, contribute to the quality and accuracy of the model. Yet, can we sustain that extracted value at the same cadence by continuing the size increase? Or will the curve start to plateau at some point?
By Nick Kucharewski, Senior Vice President and General Manager, Cloud Platform Business Unit, Marvell
The rapid expansion in the size and capacity of AI workloads is significantly impacting both computing and network technologies in the modern data center. Data centers are continuously evolving to accommodate higher performance GPUs and AI accelerators (XPUs), increased memory capacities, and a push towards lower latency architectures for arranging these elements. The desire for larger clusters with shorter compute times has driven heightened focus on networking interconnects, with designers embracing state-of-the-art technologies to ensure efficient data movement and communication between the components comprising the AI cloud.
A large-scale AI cloud data center can contain hundreds of thousands, or millions, of individual links between the devices performing compute, switching, and storage functions. Inside the cloud, there is a tightly ordered fabric of high-speed interconnects: webs of copper wire and glass fiber each carrying digital signals at roughly 100 billion bits per second. Upon close inspection there is a pattern and a logical ordering to each link used for every connection in the cloud, which can be analyzed by considering the physical attributes of different types of links.
For inspiration, we can look back 80 years to the origins of modern computing, when John von Neumann posed the concept of memory hierarchy for computer architectures1. In 1945 Von Neumann proposed a smaller faster storage memory placed close to the compute circuitry, and a larger slower storage medium placed further away, to enable a system delivering both performance and scale. This concept of memory hierarchy is now pervasive, with the terms “Cache”, “DRAM”, and “Flash” part of our everyday language. In today’s AI cloud data centers, we can analyze the hierarchy of interconnects in much the same way. It is a layered structure of links, strategically utilized according to their innate physical attributes of speed, power consumption, reach, and cost.
The hierarchy of interconnects
This hierarchy of interconnects provides a framework for understanding emerging interconnect technologies and to assess their potential impact in the next generation of AI data centers. Through a discussion of the basic attributes of emerging interconnect technologies in the context of the goals and aims of the AI cloud design, we can estimate how these technologies may be deployed in the coming years. By identifying the desired attributes for each use case, and the key design constraints, we can also predict when new technologies will pass the "tipping point" enabling widespread adoption in future cloud deployments.
By Kirt Zimmer, Head of Social Media Marketing, Marvell
What do you get when you combine some of the world’s leading technology analysts with incredibly smart subject matter experts? Answer: the SixFive Media video podcast. It’s must-view content for anyone interested in understanding exactly how AI technologies are evolving.
At Marvell’s recent Investor Analysts Day, company leaders were happy to chat with Patrick Moorhead, CEO and Chief Analyst at Moor Insights & Strategy, and Daniel Newman, CEO and Chief Analyst at The Futurum Group. The resulting conversations (captured on video) were enlightening:
How Custom HBM is Shaping AI Chip Technology
Fresh off Marvell’s announcement of a partnership with SK Hynix, Micron Technology and Samsung Semiconductor, Patrick and Daniel dove into the details with leaders from those organizations. The partnership centers around custom high bandwidth memory (HBM), which fits inside AI accelerators to store data close to the processors.
Custom designs alleviate the physical and thermal constraints traditionally faced by chip designers by dramatically reducing the size and power consumption of the interface and HBM base die. Marvell estimates that up to 25% of the real estate inside the chip package can be recovered via customization.
Will Chu, SVP and GM of Custom Compute and Storage at Marvell, says the company estimates that the total addressable market (TAM) for data centers in 3-4 years is $75B. Last year it was $21B. Out of that, Marvell estimates that $40-43B is for custom accelerators.
Attached to that is custom HBM, which alleviates bottlenecks for AI workloads. In Dong Kim, VP of Product Planning at Samsung Semiconductor said, “Custom HBM will be the majority portion of the market towards the 2027-28 timeframe.” As Patrick Moorhead said, “The rate of change is phenomenal.”
By Kirt Zimmer, Head of Social Media Marketing, Marvell
Marvell’s business is accelerated infrastructure for the AI era, which is a fast-evolving space that can occasionally confuse even the most earnest student. To help you keep up, we’ve partnered with VentureBeat to explore a multitude of content about that subject:
By Michael Kanellos, Head of Influencer Relations, Marvell
Computer architects have touted the performance and efficiency gains that can be achieved by replacing copper interconnects with optical technology in servers and processors for decades1.
With AI, it’s finally happening.
Marvell earlier this month announced that it will integrate co-packaged optics (CPO) technology into custom AI accelerators to improve the bandwidth, performance and efficiency of the chips powering AI training clusters and inference servers and opening the door to higher-performing scale-up servers.
The foundation of the offering is the Marvell 6.4Tbps 3D SiPho Engine announced in December 2023 and first demonstrated at OFC in March 2024. The 3D SiPho Engine effectively combines hundreds of components—drivers, transimpedance amplifiers, modulators, etc.—into a chiplet that itself becomes part of the XPU.
With CPO, XPUs will connect directly into an optical scale-up network, transmitting data further, faster, and with less energy per bit. LightCounting estimates that shipments of CPO-enabled ports in servers and other equipment will rise from a nominal number of shipments per year today to over 18 million by 20292.
Additionally, the bandwidth provided by CPO lets system architects think big. Instead of populating data centers with conventional servers containing four or eight XPUs, clouds can shift to systems sporting hundreds or even thousands of CPO-enhanced XPUs spread over multiple racks based around novel architectures—innovative meshes, torus networks—that can slash cost, latency and power. If supercomputers became clusters of standard servers in the 2000s, AI is shifting the pendulum back and turning servers into supercomputers again.
“It enables a huge diversity of parallelism schemes that were not possible with a smaller scale-up network domain,” wrote Dylan Patel of SemiAnalysis in a December article.